The success of our society is based on the human ability to communicate motives. Once intentions
overlap amongst concerned parties, they may decide to join forces and collaborate to work towards a
common goal that is beneficial to all and might not have been achieved by an individual. A group
of people or individuals with specific interests can be seen as an agent, an entity with an agency.
Most domains in the real world require collaboration to achieve higher goals and can be described
as multi-agent (MA) systems. Human intelligence is the highest observed, but we can draw further
inspiration from nature. Species like bees only survive because of their ability to communicate and
collaborate effectively. If a beehive successfully fends off predators, assigns tasks to collect nectar
from flowers, and building materials, build and repair the hive, their survival is ensured.
The last two years have brought climate change to the doorstep of many. Extreme heatwaves,
wildfires and floods make life for animals and humans increasingly difficult. While vivid wildfires
have destroyed large forest areas, cattle pasture is the biggest cause of deforestation.
Forests absorb more than a quarter of global human-emitted carbon dioxide. Droughts cause
soil erosions and turn pasture and woodland into steppe. The Sahara desert advances irreversibly
southward, causing livestock losses, human migration and mortality. The Maradi and Zinder
Regions have been regreening five million hectares to prevent further decline of the biosphere
and habitability of the Niger. Multiple parameters can make reforestation difficult and must
be considered to regreen successfully and sustainably. Trees need to be planted close to existing
woodland to shield seedlings from harsh winds and provide them with stable soil. Furthermore, a
mixture of native tree species needs to be planted rather than one kind. The additional maintenance,
monitoring and transportation challenges, especially in hard-to-reach areas have motivated this
work. A wide variety of unresembling landscape scenarios makes this especially hard and requires
coordination between multiple actors and information sources.
Core questions we ask: Can agents in a MA system learn the importance of communication and
subsequently extend each other’s partial observability? Furthermore, can agents learn to use the
communicated information to increase individual performance? Can shared information be used to
explore further and take actions with higher risks of depleting the battery for the benefit of finding
locations with a higher need for reforestation and accordingly higher rewards? Finally, can agents
organise themselves and delegate tasks, such that one group of agents become scouts and information
collectors to extend the collectives’ observable space and another group that carries tree seeds and
plants them accordingly in areas they would not have found by themselves? Trying to answer these
questions was the challenge of the experiments conducted and presented in this paper.


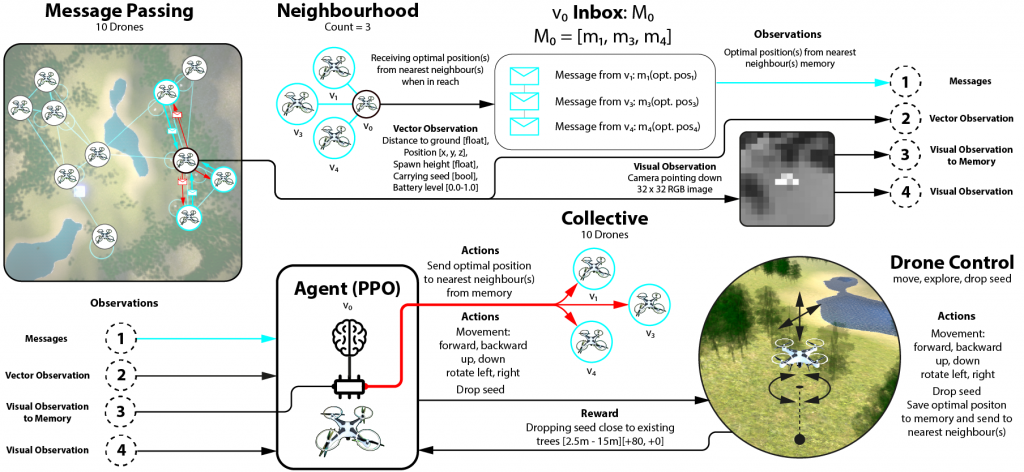
Environment Walkthrough
Poster Presentation at Neurips 2022
Drone based Reforestation Environment