Every city, neighbourhood, block and building have their own soundscape and mixture of tone. If this soundscape is perceived as noise or pleasant is a subjective matter to be decided by every individual themselves. Most of the times architects don’t specifically design sound aware, only when a client specifically asks for sound aware design considerations. In that case, a sound consultant has to be brought into the team, to give insight into how noise can be suppressed or nurtured, how much noise is bearable and what can be done with geometry and architectural building mass. Simply, architects do not have the right tools to get real-time feedback on the impact of their design on the soundscape surrounding it. Sound samples have been captured with professional hardware to register the sound pressure at specific locations, which have been related to distances to geometrical condition, traffic frequency of streets and train tracks as well as natural elements like trees, which might be home to birds and wildlife. A neural network has been utilised and trained using a set of hyperparameters consisting of adjacencies and distances, which could then be used to predict the soundscape for a fictive new development, fed back to the designers in real-time.
City Soundscape Prediction Setup
Location

Gendarmenmarkt, Berlin Germany
Sound recording location 1
In the middle of Gendarmenmarkt, wide open space, solid sealed surface, close to high frequency traffic street.



Sound recording location 2
East Gendarmenmarkt, close to a high frequency traffic street and a group of trees.


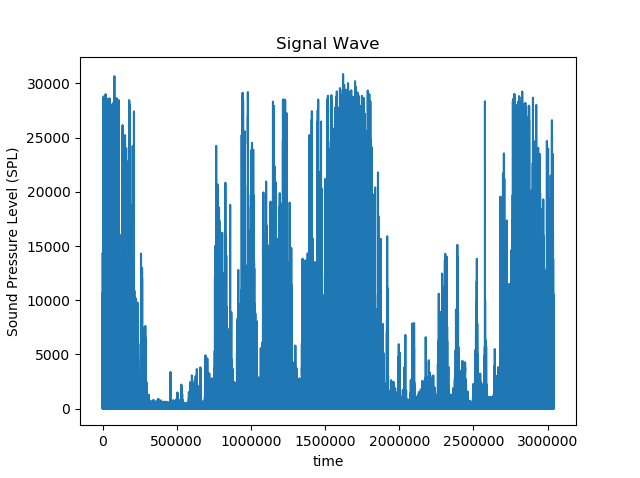
Sound recording location 3
On the south side of Gendarmenmarkt, close to medium frequency traffic street and the underground train tracks.



Hyper Parameters
Traffic as Source for Sound
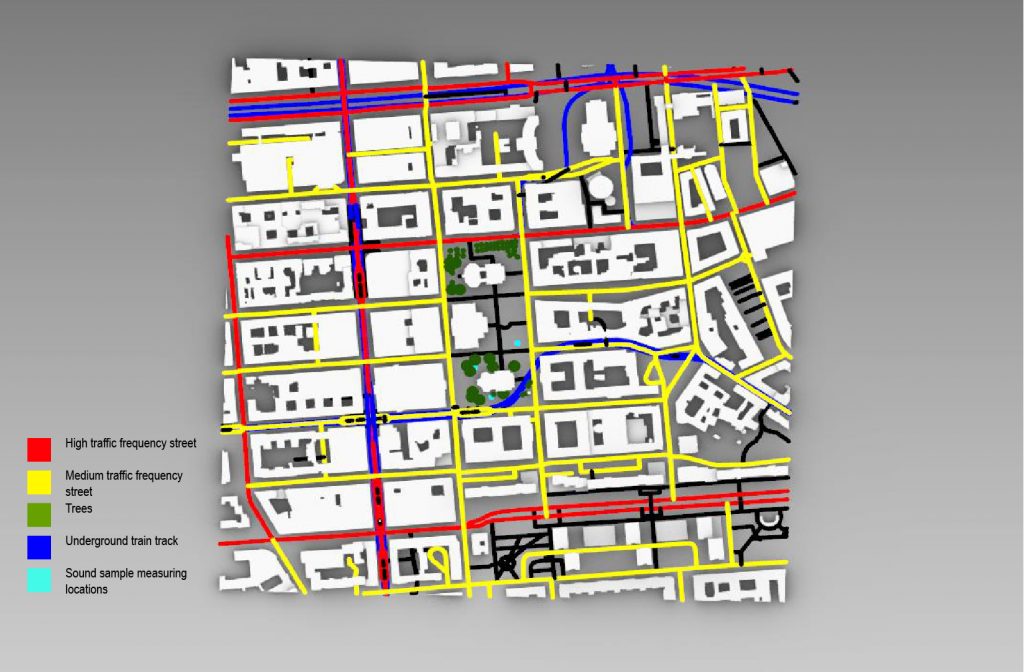
Betweenness Map

Distance from Each Pixel to Sources of Sound and Geometry

Soundscape Interpolation using Locally Captured Sound Samples

Predicting Soundscape in Fictive Design Szenario
Traffic as Source for Sound

Betweenness Map

Soundscape Prediction using Trained Neural Network
